As you may know, AI is tremendously reshaping entire industries and businesses. One technology that has received a lot of consideration is AI chatbots such as ChatGPT, capable of automating processes within education, content generation, as well as customer service. But, like any other technology, there are concerns because the results and efficiency of an AI model are only as good as the data it was trained on. By examining how bias seeps into the training data, it becomes apparent why ChatGPT isn’t able to provide fair and accurate resolutions at times. If we move toward closing these gaps, we will be able to build AI models that are less harmful. In this article we will redefine the problem and propose ways in which AI systems can be made less biased.
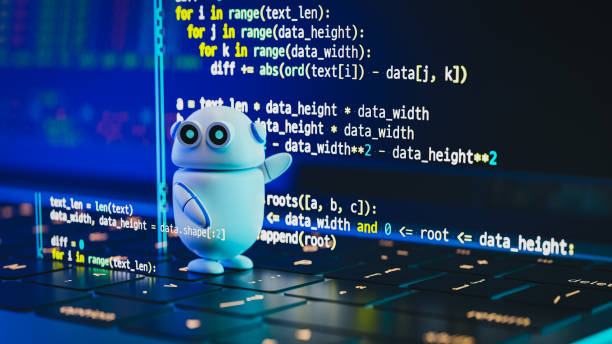
Systems like ChatGPT operate through automatic language recognition, which requires extensive training datasets to understand and respond to human languages. These datasets are not random text; they are sorted text files that represent the culture and knowledge of society at the time the text was written, which contributes to the effectiveness of the AI. The AI algorithm will then analyze this information to detect associations, linguistic features, and other contextual information within the data. This emphasizes that if the training data is not of good quality, the chances that an AI will respond accurately are significantly reduced. Nevertheless, this can become problematic if the data is prejudiced because the result will invariably be incorrect. This makes the life of developers more complex because they require frequent engagement with the data sets.
Varied training data is key to minimizing biases in artificial intelligence. A diverse dataset incorporates different cultures, languages, and perspectives, allowing the model to learn in a meaningful and contextual way. In the absence of such diversity, AI faces the danger of overly myopic views, ignoring the intricacies of human language. As an example, consider the following crucial elements for designing a balanced training dataset:
- Inclusion of voices from various racial and ethnic groups.
- Representation of different genders and sexual orientations.
- Variety in socio-economic backgrounds and geographical locations.
The AI’s efficiency is improved with such a diverse range, ensuring that no stereotypes or biases within society are accidentally supported. The results of this effort might create a more equitable and amiable AI system, where all users feel recognized.
Types of Bias in AI
For both users and developers, understanding the various types of underlying AI biases is very important. These biases are often hidden within systems or models like ChatGPT and can decrease the overall effectiveness of the model. Below are two major biases that frequently occur:
Representation Bias
When certain groups are underrepresented in training data, it can make AI comprehension and response difficult for those groups. Users trying to receive help or information may face real-life consequences because of this. The alienation felt due to the lack of proper representation raises suspicions regarding AI tools. This can further widen the existing digital gap and may deeply erode trust in technology.
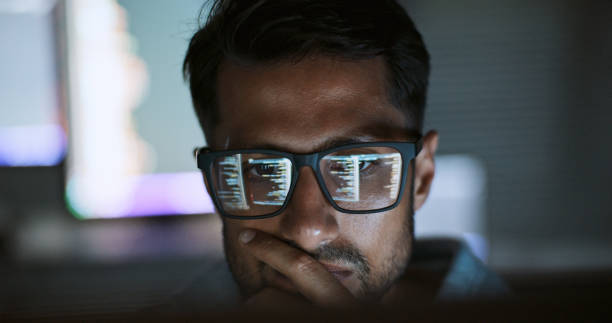
Confirmation Bias
Confirmation bias is yet another concern. AI systems, when taught exclusively on popular opinions and facts, tend to feature a single-minded perspective. This bias restricts the variety of information provided to users, causing certain ideologies to be favorably accepted over others. As a consequence, the ability to actively grapple with different viewpoints, which is vital for sound judgment, is diminished. Consider the possible consequences of confirmation bias:
- Reinforcement of existing stereotypes or ideologies.
- Limited critical engagement with alternative or dissenting opinions.
- Failure to provide comprehensive responses to user queries.
The Impact of Bias on ChatGPT’s Performance
The biases ingrained within the training data can greatly hinder the efficiency of ChatGPT. In the search for accurate information and sophisticated comprehension, users are left with unrefined and misleading answers. Due to the different contexts that ChatGPT struggles to accommodate, this can result in dire misinterpretations. Below is a table that demonstrates the correlation of the different types of biases and the likely impacts on performance:
Type of Bias | Potential Effect |
---|---|
Representation Bias | Inability to understand or respond to diverse users. |
Confirmation Bias | Narrow perspectives, missing out on alternative viewpoints. |
Such biases can undermine trust in AI systems, especially in sensitive fields like education. The negative effects of biases may reduce trust and satisfaction, leading AI users to lose credibility in the system. This is far more worrisome in education where, under misinformation, students are likely to learn less and become less involved.
Strategies for Mitigating Bias
Tackling bias in AI systems effectively demands multi-dimensional approaches that are the responsibility of developers, users, and other stakeholders. These approaches aim to establish an AI system that is representative of experiences and opinions from multiple sectors. Some of the primary methods are:
- Model Refinement: Continuous refinement of AI models to ensure they adapt to new information and cultural shifts is crucial. Developers must update training datasets consistently to include marginalized voices and underrepresented perspectives.
- User Feedback Mechanisms: Implementing robust feedback systems allows users to highlight biased outputs, which can help developers make ongoing adjustments and improvements. Such feedback loops also create a sense of community engagement around AI tools.
- Comprehensive Testing: Rigorous testing of AI tools across various demographic scenarios can help identify biases proactively and mitigate them before reaching the end-user.
These strategies not only refine the AI model’s capabilities but also foster inclusivity in AI interactions.
Conclusion
The biases existing in AI, especially in systems such as ChatGPT, present great problems with regard to their accuracy and efficacy. These biases can, in one way or another, impact people negatively. If addressed properly, these limitations can allow us to create a fairer AI world. Talking is not enough; action must be taken for the models to truly change and incorporate diverse viewpoints. With technological change comes responsibility in ensuring that inclusivity becomes an integral part of AI implementation in sensitive areas such as education.
FAQ
- What is bias in AI? Bias in AI refers to systematic errors that can lead to unfair outcomes, often stemming from unbalanced training data.
- How does bias affect ChatGPT? Bias can lead to inaccurate outputs, particularly in representing marginalized voices or perspectives.
- What contributes to bias in AI training data? Bias often arises from underrepresentation of certain demographics, historical injustices, and prevalent societal stereotypes in the data.
- Can bias in AI be completely eliminated? While it may not be possible to completely eliminate bias, ongoing refinements and diverse training sets can significantly reduce it.
- How can users identify biased outputs from AI? Users can look for inconsistencies or negative stereotypes in responses and seek diverse perspectives to cross-check information.